If there’s one thing that grinds Amanda Richardson’s gears, it’s fads.
As Chief Data and Strategy Officer for HotelTonight (she was previously their VP Product), she oversees 400 to 500 data events for an app with millions of monthly users. Her team registers hundreds of events from every user session. She’s steeped in the data world and is a keen observer of how other companies are increasingly leveraging data to make decisions. But what she sees are a number of trends and approaches to data that aren’t just lazy, but also ineffective, misleading, and costing companies a ton of opportunity.
In this exclusive interview, Richardson points out four of these approaches that data teams industry-wide should watch out for, and how leaders can break from the pack to better serve their strategic goals. Specifically, she describes how she built her current team to mimic the product teams she's led for years. And she explains why creating next-generation products has a lot more to do with simplicity, consistency and consensus than leveraging the hottest new thing.
Mistake #1: Starting with Metrics Instead of a Goal
Data is more available than ever before. Collecting, storing and analyzing it have become standard practices at most companies. But all too often, lost in the scramble for real-time analytics and data lakes is a coherent and straightforward plan for what to do with all of these numbers. Richardson has seen many a team aimlessly digging through data, looking for the story. With no clear point of view, though, they’re left chasing a moving target.
“You need to start with a specific question to answer or hypothesis to investigate,” she says. “A lot of times, people will launch a product and then say, ‘How's it doing?’ Rather than saying, ‘Our product goal is to convert from this to this. Or grow the top of funnel or move the bottom of the funnel. Or increase our revenue per user.’”
Without a clear and agreed-upon goal, you run the risk of rewriting history as the data comes in. Take HotelTonight’s Favorites Feature that allows users to 'favorite' hotels they want to call up first during searches. “Is that for our power users? Is it for new people who may not like the serendipity of HotelTonight and want to follow a specific hotel? Is it for people planning a trip who want a watchlist feature?” says Richardson. “Those are all possibilities. But what's the key metric that's going to determine success? Without that clarity, you end up with a situation where one person is saying, ‘This was great for our most popular users, who favorite an average of 12 hotels’ and another is saying ‘But this was intended for new users.’ And you’re thinking, ‘Was it?’”
The key to effectively consuming data is to clearly stipulate what you’re trying to accomplish and how you’ll define success. But that’s easier said than done. “Everyone agrees at 60,000 feet. But once you take it into the details — 'What are we going to high-five over in 30 days?' — people are not clear.”
So make things clear. Create the scorecard by which you’ll judge success. “The discipline is to have something written down before every project gets started.” Richardson cites a classic shorthand for effective goal-setting. “It’s that old SMART acronym: Specific, measurable, achievable, relevant, and timely.”
When it comes to setting that all-important goal, she’s unequivocal: That’s one of the key responsibilities of a startup’s founders. “It’s the job of the leader to say, ‘We're going to climb Everest.’ It's the job of the team to figure out the best path up Everest and the requirements for each team member. But particularly at an early-stage company, it’s not fair to leave it to the product manager to set these strategic priorities.”
Putting your goal down on paper is also the best way to resist the tendency to shift gears as you go. “It’s so easy to think, ‘Adding whatever feature I heard my buddy mention at a dinner party last night is all of a sudden my goal, too.’” It’s also easy to take wins wherever you find them. “We all want to be right. Maybe you said, "Our goal is to grow first-time users by 10%,’ then you see that feature is used by repeat users 30%. All of a sudden you're saying, ‘That's a big win! We got 30% of repeats!’ People lose sight of their goal all the time.”
It’s easy to understand why. “There's so much pressure. CEOs need to demonstrate success. If the team is growing, they’ve got this new family of mouths to feed, and it’s very stressful. But it's their job, more than anyone, to be honest about what the goal is.”
Be as precise as possible about your top-level objective, and the metrics you’re using to measure it. “It might be ‘Our big goal is to get this many bookings of this type by this date.’” Be clear, too, about the number that represents success.
That’s not to say that you have to turn a blind eye to other insights that surface in your data. But keep your focus trained on what you set out to accomplish and the data you came for. “When you ask data people how a product or feature is going, they’ll almost always come back with a list of fun facts,” says Richardson. “But don’t confuse those secondary metrics with your top metric.” Not sure where to start? Take it a quarter at a time.
Think about your number one priority for the quarter. Do you have a clear, definable metric around it?
Mistake #2: Rampant Personalization
For many startups, personalization — customizing a user’s home screen, for example, or offering recommendations — is a solution in search of a problem. It’s a hallmark of hugely successful companies like Amazon (recommended products) and Facebook (a curated Newsfeed), so it stands to reason that it’s a good thing, right?
Not necessarily, and definitely not necessarily right now. As with every decision early leaders make, you have to think about opportunity cost. “Personalization takes a ton of development time,” says Richardson. “In many ways, it’s just another feature. Is it one you even need, or one that makes sense for your product?”
If the answer is yes, you may still need to hold off. Effectively personalizing a product generally requires a good amount of banked data — data that a new company may not have had time to amass.
“I’ve talked to people at pre-launch startups with fewer than 100 users who say, ‘We’re going to start on personalization,’” says Richardson. “And I’m thinking, ‘What are you going to personalize? And more importantly, why? What is the problem you’re solving?’ Often the motivation is that it’s in a headline or a board member thinks it’s the secret to success.”
It’s not that she’s some anti-personalization crusader — tailored results are a key element of HotelTonight’s user experience. But it took a lot of data to understand how those users wanted personalization to work. Ultimately, the company realized that travelers didn’t want to see suggestions based simply on history, but on context.
“Maybe my last booking was a Luxe hotel. It was the weekend. It was my anniversary,’” says Richardson. “But if I show up in New York City for work and book a Luxe, that expense report is getting rejected. Day of week matters. Time of day matters. If you're booking at 11 o'clock at night, you really don't care if they have a spa, right? All of those factors, which are personalization, matter far more than the individual user. We wouldn’t have seen this if we would have rushed our efforts to personalize the experience.”
There’s no single answer to if and when you should undertake personalization. Richardson’s recommendation is, once again, simply to start with a goal, or a hypothesis to test. “Tell me what's not working. Then maybe personalization is a solution,” she says. “Let’s say conversion in New York City's down, and we don't know why. Maybe a solution is to personalize the display of hotels to you. But it better be a pretty good bet to merit the time and human cost.”
For some startups, personalization may be closely tied to mission and need to come early. For others, it may never be worth the investment. “If you’re a new photo stream app, the time to pursue this is probably sooner because it can be a core differentiator. If you're selling B2B expense software, it's probably never.”
Focusing too much on personalization has steep opportunity cost. It’s always a tradeoff with real development time. When you don’t waste your time going down rabbit holes, you can use it to pursue more important growth opportunities with a higher likelihood of success, says Richardson. Your data team can work on answering business critical questions instead — like getting a better sense of what drives users to convert, which you might think personalization will solve, even if it doesn’t.
Until you can identify the problem you're solving, stop throwing around solutions because they're cheap and catchy.
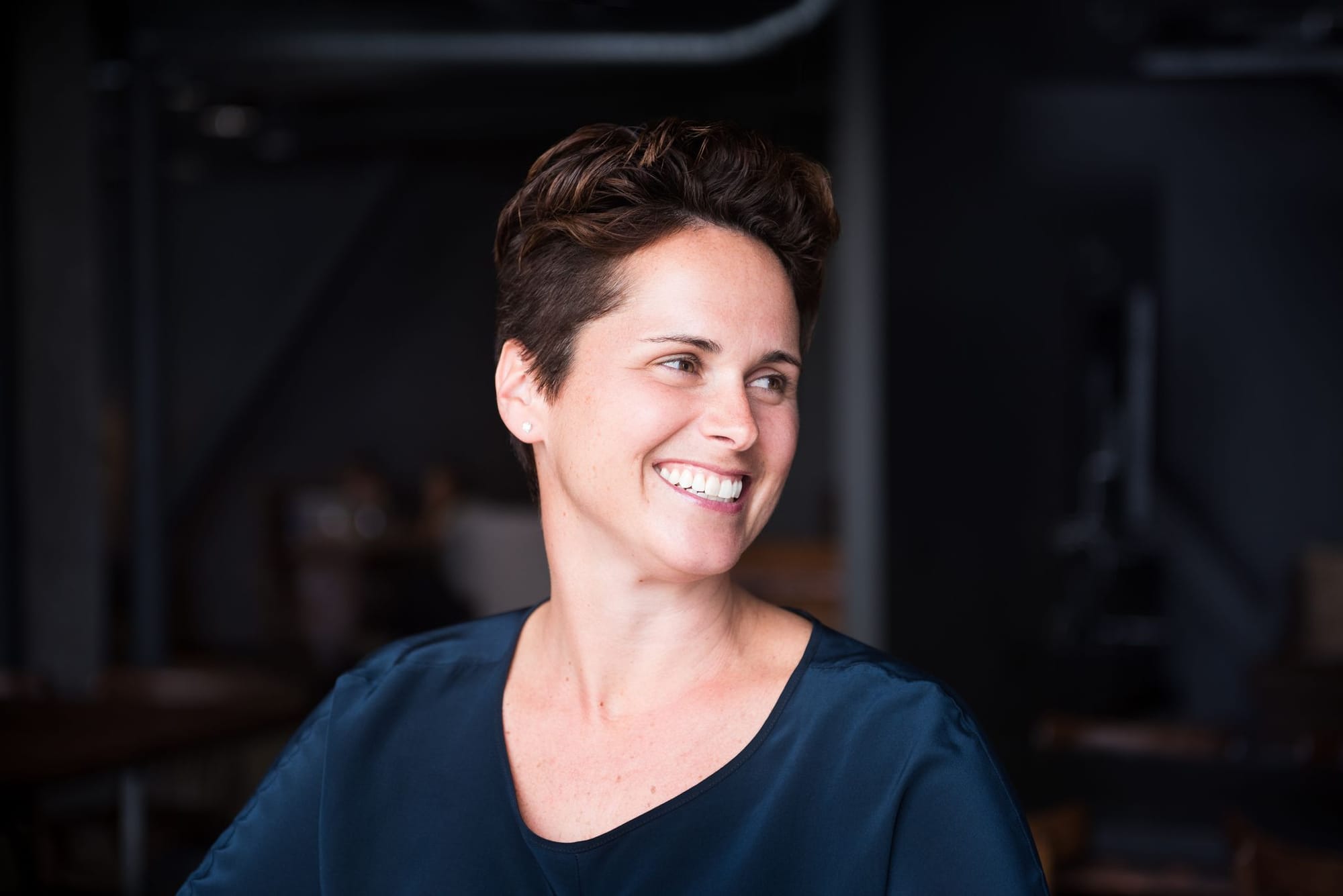
Mistake #3: Hiring a Dedicated Data Scientist
If you think that your organization’s analysis needs to come in the form of a dedicated employee with a “data scientist” title, Richardson challenges you to think broader. “To me, data science is a collection of skills, not a job. Just like I would say analysis and strategy is a collection of skills, not a job. Everybody on your early team needs to be strategic. Everybody should be able to do analysis.”
She cites the recent movie Hidden Figures, the story of the women mathematicians behind NASA’s early missions. “The women were called ‘computers,’ right? They used to be the only people dedicated to doing complex calculations. But that’s not a job anymore — now everyone does computations — it’s become part of a generalized skill set. That’s how the world evolves. And I think we’re there with data science. More people should be able to take responsibility for and have the capability to work with data to make decisions.”
Think about what effective data science requires: There’s the statistical, computational chops, of course. But it also demands enough knowledge of the market, and how your business functions within it, to ask the right questions and meaningfully answer the those questions. Also critical are strong programming skills. Too often, though, companies hire for the stats skills only, then silo that person so they can never access the business context they need. This isolation blinds the data scientist to the realities of how the company is operating. And, it makes their results and recommendations theoretical and often irrelevant.
“Our mistake — and the mistake I've seen often — has been to hire a data scientist who is very technical, but who lacks business acumen. Then we isolate that person and have them churn through the million questions we inevitably want to answer.” However skilled that person might be, they don’t have the context to be successful. And they don’t have time or opportunity to interact with the engineers, PMs, or marketing leads that could give it to them. “They don't know what to look for, and they aren’t empowered to ask the right questions,” says Richardson.
“One of my favorite examples of this at HotelTonight is that data scientists will often come in and remark, ‘"The people who become our highest value users are people who don't convert in the first two weeks. They convert in days 15 to 30 after installment.’ Which is true. But what am I going to do with that? It’s a fun fact.”
Meanwhile, a lot of good questions are probably getting stuck in the queue. “People wait in line for the data scientist's time rather than saying, ‘How can we figure this out ourselves?’” says Richardson. “You’ll be much more successful if you create an organization where everyone feels empowered and educated on how to do analysis.”
Rather than focusing on an overly narrow title, or a single job req, consider the skills a data scientist brings to bear. Odds are good you already have them on board. “People say, ‘I can't afford a data scientist,’ but that’s crazy. Someone on your team took a stats class. I hope you have a developer. And someone should have business acumen — the CEO, if no one else. Get those three people in a room. You don’t have to wait for this magic person to appear.”
Moreover, startups should think twice about whether they even have enough material for a pricey data scientist to work with. “To do deep analysis, you need a lot of data. If it's your third month as a start up, and you've got 60 users, there's probably not enough data.”
If and when you do hire a dedicated data scientist, don’t stick them in a corner. Include them in the meetings and conversations that will inform how you apply the numbers they’re crunching. “I’m reflecting on how we failed with our data scientist the first time,” says Richardson. “For starters, he literally sat by himself away from all of us.” Everyone on your team works better and smarter when they understand their role as part of a whole. Even if you have to do some nudging, get your numbers-minded data people out of their comfort zone and into the business.
You should always be looking to build out engineering skills, statistical knowledge, and business acumen — and support those employees who strive to exhibit the full suite. But especially early in your company's life, you’re typically better served to hire three experts and make them come together on analysis than you are to look for one unicorn who can do all of those things.
Mistake #4: Chasing After the Latest Toolset
New tools emerge all the time. But at the end of the day, they can’t make your data strategy fool proof or perfect. “Garbage in, garbage out,” says Richardson. “A tool can absorb whatever events you want to send it, but it’s you that has to define what those events are and what they mean. And you have to make sure those events don’t change.”
There’s no substitute for clarity and consistency, nor a tool that can replicate a clear point of view. Meanwhile, even the simplest tools can be an effective way to manage data if you apply the right rigor — and early stage companies with burn rate on the brain should take heed. There are three things that Richardson recommends every data team supply their organizations:
- A central dashboard: “This goes back to the first point. When you know what your top-line metric is and you put it on a dashboard, then no one else can change it. If it's the bold headline at the top, then no one can come back and say, ‘That wasn't our goal.’” What you use can be as simple as a locked Google Doc. The idea is simply to have a canonical source of truth for what you’re doing, as well as a resource that you can push out to the rest of the organization on a regular basis.
- Accessible data: If you want everyone on your team to be empowered to think analytically, you need to give them easy access to the numbers. “For marketing that may be your attribution stuff. For product, that's your conversion stuff. For engineering, maybe your uptime.” The most important thing is simply to encourage hands-on engagement with each person’s part of the business. “The good news is there’s now a whole generation of people who have grown up with computers and access to anything they want, whenever they want it.”
- Flexible tooling: Adoption of that mindset, though, is a lot more important than adoption of any single tool. “Just let everybody have their own specialized data tool. There is no one solution.” Her team uses Looker; other teams might find that something else works better for their analytics. “I hear people say, ‘Wouldn't it be great if we all used Looker for everything?’ There's no amount of time in the world for me to build enough Looker capabilities, even customizations, to answer questions as well as, say, Amplitude for the product team. So let them use the tool that works for them.”
Why You Should Run Data Like You Run Product
Richardson can’t predict the next data trend coming down the pike, but she has found a way to make sure her team isn’t distracted by the latest shiny object: she runs HotelTonight's data operations like a product team.
Three years into her tenure as VP of Product for HotelTonight, cofounder Sam Shank asked her to lead the company’s data efforts. “We’d gotten to a point where it became clear that people didn’t agree on what our core metrics were, and he wanted to fix that,” she says. “What I immediately saw was the same problem you have in product: a lot of stakeholders, with a lot of different opinions about what is most important.”
That’s the bad news. The good news? Well, data teams suffer from the same problems that product teams do—so there’s a roadmap of processes and best practices there for the taking.
Richardson saw that she needed to build the same type of infrastructure she did as a product leader. “We need a backlog. We need someone who knows what the solution is. We need someone who's talking to our users about how they use data, what they want to use data for. We need a QA process to make sure we don't change the data.”
First things first, she made sure that just as someone owns a product, someone owned data product. “I have an amazing director of data product, who’s literally a PM by training. He works across the organization to figure out how teams are using data. He prioritizes. He does sprint planning with the data engineering team every week," says Richardson.
Next, the team is responsible for ensuring the data coming in is good, which they do with a rigorous QA test suite. “We’re always monitoring and automating the data in the background, so that we don’t realize something’s broken after three weeks. ‘Did the data change from day to day? Is the data abnormally out of bounds? Is there no data coming in?’ All the same kind of product-behavior questions you would ask on the product side.”
One thing Richardson has found more central to her work in data than her previous product role is documentation. To achieve crisply defined goals, you need to work with a shared vocabulary, and her team is responsible for defining everything from “revenue” to “visit.” “Okay, a user. Does a user have to do a search? Or if someone just checks on their bookings, is that a user? Is it someone for whom we have an email address?”
Analysis succeeds or fails on these details, but reaching consensus on what these terms mean can be complicated. “Another reason to have a product owner of the data, who can work across the organization like a PM would.”
Finally, there’s deprecation. Just as maintaining a healthy product means periodically pruning features, maintaining a healthy data product requires deprecating data streams that are no longer relevant and removing them from the database. “Otherwise, someone pulls the wrong data, and you end up with seven different definitions of revenue.”
If your company is still incredibly young or small, you can still pursue this strategy. Start with one strong data engineer who cares about the business and proving value, then make that person prioritize what to go after. This person might have to wear a few hats and take on other development or analyst responsibilities to justify their hire, but they should be encouraged to think ruthlessly like a PM and learn those skills on the job.
For Richardson, there’s been one clear sign that she’s on the right track: “No one asks if the data is right anymore. They may not like the data, but no one is questioning its accuracy.”
That confidence has been the biggest payoff of Richardson’s product-based approach to data. With sharp definitions and well-oiled process, she has not only clarified the company’s goals, she’s freed the company to experiment more productively, too. “In product, you have your committed core features, and then you have the things you’re testing in MVP," she says. "We have the same concept in data. There’s the data that we’ve got the gold standard on, and then there’s new data that we’re taking in even though we’re not sure what to do with it yet.”